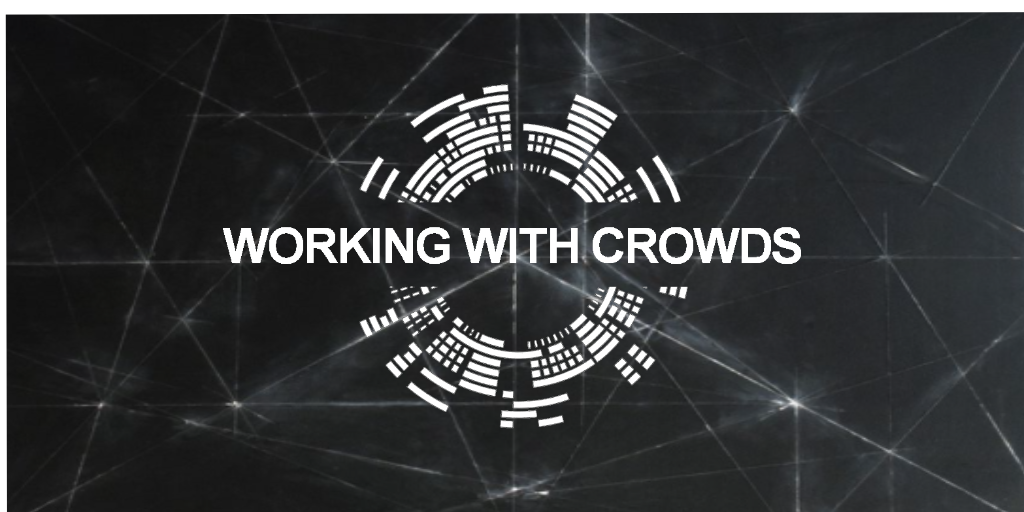
CONTENT
EVACUATION PLANNING
CROWD MONITORING/ COUNTING
ONLINE JOURNAL (JCSSM)
Crowd Monitoring /Counting
Crowd control by multiple cameras
L.J.M. Rothkrantz, Z.Yang
Abstract
One of the goals of the crowd control project at Delft University of Technology is to detect and track people during a crisis event, classify their behavior and assess what is happening. The assumption is that the crisis area is observed by multiple cameras (fixed or mobile). The cameras sense the environment and extract features such as the amount of motion. These features are the input to a Bayesian network with nodes corresponding to situations such as terroristic attack, fire, and explosion. Given the probabilities of the observed features, by reasoning, the likelihood of the possible situations can be computed. A prototype was tested in a train compartment and its environment. Forty scenarios, performed by actors, were recorded. From the recordings the conditional probabilities have been computed. The scenarios are designed as scripts which proved to be a good methodology. The models, experiments and results will be presented in the paper.
CROWD ANALYSIS USING VISUAL AND NON-VISUAL SENSORS, A SURVEY
Muhammad Irfan, Lucio Marcenaro, Laurissa Tokarchuk
ABSTRACT
This paper proposes a critical survey of crowd analysis techniques using visual and non-visual sensors. Automatic crowd understand- ing has a massive impact on several applications including surveil- lance and security, situation awareness, crowd management, public space design, intelligent and virtual environments. In case of emer- gency, it enables practical safety applications by identifying crowd situational context information. This survey identifies different ap- proaches as well as relevant work on crowd analysis by means of visual and non-visual techniques. Multidisciplinary research groups are addressing crowd phenomenon and its dynamics ranging from social, and psychological aspects to computational perspectives. The possibility to use smartphones as sensing devices and fuse this in- formation with video sensors data, allows to better describe crowd dynamics and behaviors. Eventually, challenges and further research opportunities with reference to crowd analysis are exposed.
Evaluation of pedestrian flow characteristics across different facilities inside a railway station
Monalisa Patra, Eswar Sala, K. V. R. Ravishankar
Abstract
Rail networks play major role in transporting people at any level of development of a country. Railway stations infrastructures are to be well designed and managed for platform changing and evacuation. A detailed study is to be made in understanding pedestrian flow characteristics on horizontal and vertical movement facilities. In this paper, passageway with and without centre rail, stairway and escalator are evaluated and compared within a railway station for a keen insight in variation of pedestrian traffic characteristics. Pedestrians speed on stairway is higher than on passageway. Walking speed of younger is greater than middle aged and elders have less speed. Pedestrians with luggage walk slower than pedestrians without luggage. Significant differences in speed exist with respect to attributes age, luggage, gender and direction. It is interesting to observe that the flow on passageway with and without centre rail is same. Pedestrians tend to move and merge with flow to the adjacent side of passageway connected at the neck of stairway.
Geometric and Physical Constraints for Head Plane Crowd Density Estimation in Videos
Weizhe Liu, Krzysztof Lis, Mathieu Salzmann, Pascal Fua
Abstract.
State-of-the-artmethodsofpeoplecountingincrowdedscenes rely on deep networks to estimate people density in the image plane. Perspective distortion effects are handled implicitly by either learning scale-invariant features or estimating density in patches of different sizes, neither of which accounts for the fact that scale changes must be consis- tent over the whole scene. In this paper, we show that feeding an explicit model of the scale changes to the network considerably increases perfor- mance. An added benefit is that it lets us reason in terms of number of people per square meter on the ground, allowing us to enforce physically- inspired temporal consistency constraints that do not have to be learned. This yields an algorithm that outperforms state-of-the-art methods on crowded scenes, especially when perspective effects are strong.
A texture based mani-fold approach for crowd density estimation using Gaussian Markov Random Field
Sonu Lamba, Neeta Nain
Abstract
The objective of this work is to leverage the clues obtained from mani-fold sources, to figure out the density of people existent in exceptionally dense crowded regions. The complications in crowd density estimation include perspective effect, occlusion, clutter background, textured brick surface, and few pixels per target. In crowd scenarios with these complications, detection based techniques are not accurate, even none of the single feature alone is suitable to estimate crowd density. Therefore, our methodology depends on mani-fold sources such as head detection with low confidence, recurrence of texture elements by using frequency domain, wavelet and scale invariant feature transform (SIFT) descriptor to measure the density count. The information obtained from manifold sources is used to train a support vector machine (SVM), which generates a patch count estimation. Next, a Gaussian-based Markov Random Field (MRF) is applied on image patches to obtain uniformity on crowd count. The Gaussian MRF furnishes the discrepancy in crowd count along with local neighborhoods at multiple scales. We tested our approach on four different datasets such as Shanghai Tech_A, UCF_CC_50, extended UCF_CC_100 and UCSD. The former three datasets are a crisp contrast to existing crowd datasets used in literature which contains almost hundreds or tens of individuals in crowd images. The latter UCSD dataset is used to test the robustness of our technique in low-density crowd too. We compare the proposed method with both traditional and convolutional neural network (CNN) based approaches. Low computational complexity indicates that the proposed technique provides decent performance rate and can be employed in real-world applications. Our experimental results validate the adequacy and efficiency of the intended methodology by measuring the density of crowd images.
A-CCNN: ADAPTIVE CCNN FOR DENSITY ESTIMATION AND CROWD COUNTING
Saeed Amirgholipour Kasmani, Xiangjian He, Wenjing Jia, Dadong Wang, Michelle Zeibots
ABSTRACT
Crowd counting, for estimating the number of people in a crowd using vision-based computer techniques, has attracted much interest in the research community. Although many at- tempts have been reported, real-world problems, such as huge variation in subjects’ sizes in images and serious occlusion among people, make it still a challenging problem. In this pa- per, we propose an Adaptive Counting Convolutional Neural Network (A-CCNN) and consider the scale variation of ob- jects in a frame adaptively so as to improve the accuracy of counting. Our method takes advantages of contextual infor- mation to provide more accurate and adaptive density maps and crowd counting in a scene. Extensively experimental evaluation is conducted using different benchmark datasets for object-counting and shows that the proposed approach is effective and outperforms state-of-the-art approaches.
Depth Information Guided Crowd Counting for Complex Crowd Scenes
Mingliang Xu, Zhaoyang Ge, Xiaoheng Jiang, Gaoge Cui, pei Lv, Bing Zhou, Changsheng Xu
Abstract
It is important to monitor and analyze crowd events for the sake of city safety. In an EDOF (extended depth of field) image with a crowded scene, the distribution of people is highly imbalanced. People far away from the camera look much smaller and often occlude each other heavily, while people close to the camera look larger. In such a case, it is difficult to accurately estimate the number of people by using one technique. In this paper, we propose a Depth Information Guided Crowd Counting (DigCrowd) method to deal with crowded EDOF scenes. DigCrowd first uses the depth information of an image to segment the scene into a far-view region and a near-view region. Then Digcrowd maps the far-view region to its crowd density map and uses a detection method to count the people in the near-view region. In addition, we introduce a new crowd dataset that contains 1000 images. Experimental results demonstrate the effectiveness of our DigCrowd method.
On Crowd Density Estimation for Surveillance
H. Rahmalan, M.S. Nixon, J. N. Carter
Abstract
The goal of this work is to use computer vision to measure crowd density in outdoor scenes. Crowd density estimation is an important task in crowd monitoring. The assessment is carried out using images of a graduation scene which illustrated variation of illumination due to textured brick surface, clothing and changes of weather. Image features were extracted using Grey Level Dependency Matrix, Minkowski Fractal Dimension and a new method called Translation Invariant Orthonormal Chebyshev Moments. The features were then classified into a range of density by using a Self Organizing Map. Three different techniques were used and a comparison on the classification results investigates the best performance for measuring crowd density by vision
Crowd density estimation based on statistical analysis of local intra-crowd motions for public area surveillance
Zhaoxiang Zhang, Min Li
Abstract.
Crowd density estimation in public areas with people gathering and waiting has been a challenging problem for visual surveillance over many years. Tiny motions, like when people turn around, wander about, and turn their heads, happen randomly now and then in crowds, which makes it difficult to achieve high-performance crowd density estimation based on traditional foreground detection. A novel accumulated mosaic image difference feature is proposed to represent these complicated random motion patterns for accurate foreground detection. The obtained foreground is then normalized based on the perspective distortion correc- tion model to achieve a reasonable crowd density measurement for observed areas. Numerous experiments are conducted in different scenes of various view angles, and experimental results demonstrate the effectiveness and robustness of our proposed method.
Privacy Preserving Crowd Monitoring: Counting People without People Models or Tracking
Antoni B. Chan Zhang-Sheng John Liang Nuno Vasconcelos
Abstract
We present a privacy-preserving system for estimating the size of inhomogeneous crowds, composed of pedestrians that travel in different directions, without using explicit ob- ject segmentation or tracking. First, the crowd is segmented into components of homogeneous motion, using the mixture of dynamic textures motion model. Second, a set of simple holistic features is extracted from each segmented region, and the correspondence between features and the number of people per segment is learned with Gaussian Process re- gression. We validate both the crowd segmentation algo- rithm, and the crowd counting system, on a large pedes- trian dataset (2000 frames of video, containing 49,885 total pedestrian instances). Finally, we present results of the sys- tem running on a full hour of video.
A Viewpoint Invariant Approach for Crowd Counting
Dan Kong, Doug Gray and Hai Tao
Abstract
This paper describes a viewpoint invariant learning-based method for counting people in crowds from a single camera. Our method takes into account feature normalization to deal with perspective projection and different camera orientation. The training features include edge orientation and blob size histograms resulted from edge detection and background subtraction. A density map that measures the relative size of individuals and a global scale measuring camera orientation are estimated and used for feature normalization. The relationship between the feature histograms and the number of pedestrians in the crowds is learned from labeled training data. Experimental results from different sites with different camera orientation demonstrate the performance and the potential of our method
Marked Point Processes for Crowd Counting
Weina Ge and Robert T. Collins
Abstract
A Bayesian marked point process (MPP) model is developed to detect and count people in crowded scenes. The model couples a spatial stochastic process governing number and placement of individuals with a conditional mark process for selecting body shape. We automatically learn the mark (shape) process from training video by estimating a mixture of Bernoulli shape prototypes along with an extrinsic shape distribution describing the orientation and scaling of these shapes for any given image location. The reversible jump Markov Chain Monte Carlo framework is used to efficiently search for the maximum a posteriori con- figuration of shapes, leading to an estimate of the count, location and pose of each person in the scene. Quantitative results of crowd counting are presented for two publicly available datasets with known ground truth.
Multi-Source Multi-Scale Counting in Extremely Dense Crowd Images
Haroon Idrees, Imran Saleemi, Cody Seibert Mubarak Shah
Abstract
We propose to leverage multiple sources of information to compute an estimate of the number of individuals present in an extremely dense crowd visible in a single image. Due to problems including perspective, occlusion, clutter, and few pixels per person, counting by human detection in such images is almost impossible. Instead, our approach re- lies on multiple sources such as low confidence head de- tections, repetition of texture elements (using SIFT), and frequency-domain analysis to estimate counts, along with confidence associated with observing individuals, in an im- age region. Secondly, we employ a global consistency con- straint on counts using Markov Random Field. This caters for disparity in counts in local neighborhoods and across scales. We tested our approach on a new dataset of fifty crowd images containing 64K annotated humans, with the head counts ranging from 94 to 4543. This is in stark con- trast to datasets used for existing methods which contain not more than tens of individuals. We experimentally demon- strate the efficacy and reliability of the proposed approach by quantifying the counting performance.
Counting people in the crowd using a generic head detector
Venkatesh Bala Subburaman, Adrien Descamps, Cyril Carincotte
Abstract
Crowd counting and density estimation is still one of the important task in video surveillance. Usually a regression based method is used to estimate the number of people from a sequence of images. In this paper we investigate to estimate the count of people in a crowded scene. We detect the head region since this is the most visible part of the body in a crowded scene. The head detector is based on state-of-art cascade of boosted integral features. To prune the search region we propose a novel interest point detector based on gradient orientation feature to locate regions similar to the top of head region from gray level images. Two different background subtraction methods are evaluated to further reduce the search region. We evaluate our approach on PETS 2012 and Turin metro station databases. Experiments on these databases show good performance of our method for crowd counting.
An Improved Smartphone-based Non-Participatory Crowd Monitoring System in Smart Environments
Tarun Kulshrestha ,Divya Saxena ,Rajdeep Niyogi, Manoj Misra , Dhaval Patel
Abstract included in link
Noninvasive Bluetooth Monitoring of Visitors’ Length of Stay at the Louvre
Yuji Yoshimura, Anne Krebs, Carlo Ratti
The ubiquity of digital technologies is revolutionizing how researchers collect data about human behaviors. Here, the authors use anonymized longitudinal datasets collected from noninvasive Bluetooth sensors to analyze visitor behavior at the Louvre Museum.
Exploiting Density to Track Human Behavior in Crowded Environments
Claudio Martella, Marco Cattani, and Maarten van Steen
Abstract
For the Internet of Things to be people-centered, thingsneed to identify when people and their things are nearby. In this paper, we present the design, implementation, and deployment of a positioning system based on mo- bile and fixed inexpensive proximity sensors that we use to track when individuals are close to an instrumented object or placed at certain points of interest. To over- come loss of data between mobile and fixed sensors due to crowd density, traditional approaches are extended with mobile-to-mobile proximity information. We tested our system in a museum crowded with thousands of vis- itors, showing that measurement accuracy increases in the presence of more individuals wearing a proximity sensor. Furthermore, we show that density information can be leveraged to study the behavior of the visitors, for example, to track the popularity of points of interest, and the flow and distribution of visitors across floors.
Analysis of pedestrian behaviors through non-invasive Bluetooth monitoring
Yuji Yoshimura, Alexander Amini, Stanislav Sobolevsky, Josep Blat, Carlo Ratti
Abstract
This paper analyzes pedestrians’ behavioral patterns in the pedestrianized shopping environment in the historical center of Barcelona, Spain. We employ a Bluetooth detection technique to capture a large-scale dataset of pedestrians’ behavior over a one-month period, including during a key sales period. We focused on comparing particular behaviors before, during, and after the discount sales by analyzing this large- scale dataset, which is different but complementary to the conventionally used small- scale samples. Our results uncover pedestrians actively exploring a wider area of the district during a discount period compared to weekdays, giving rise to strong underlying mobility patterns.
Tracking Pedestrians and Emergent Events in Disaster Areas
Gu ̈rkan Solmaz, Damla Turgu
Abstract
Most of the existing research on emergency evacuation strategies focus on city evacuation planning that highly depends on the use of vehicles or evacuation from buildings. However, for large areas with limited use of vehicles such as theme parks, evacuation of pedestrians and emergent events must be tracked for safety reasons. As hazards may cause certain damages to services, networks with disaster resilience are needed to achieve mission- critical operations such as search and rescue. In this paper, we develop a method for tracking pedestrians and emergent events during disasters by opportunistic ad hoc communication. In our network model, smart-phones of pedestrians store and carry messages to a limited number of mobile sinks. Mobile sinks are responsible for com- municating with smart-phones and reaching the emergent events effectively. Since the positioning of the mobile sinks has a direct impact to the network performance, we propose physical force based (PF), grid allocation based(GA) and road allocation based (RA) approaches for sink placement and mobility. The proposed approaches are analyzed through extensive network simulations using real theme park maps and a human mobility model for disaster scenarios. The simulation results show that the proposed approaches achieve significantly better network coverage and higher rescue success without producing increased communication overhead compared to two random mobile sink movement models.
Beyond Counting: Comparisons of Density Map for Crowd Analysis Tasks – Counting, Detection,and Tracking
Di Kang, Zheng Ma, Student Member, IEEE, Antoni B. Chan Member, IEEE
Abstract
For crowded scenes, the accuracy of object-based computer vision methods declines when the images are low- resolution and objects have severe occlusions. Taking counting methods for example, almost all the recent state-of-the-art counting methods bypass explicit detection and adopt regression- based methods to directly count the objects of interest. Among regression-based methods, density map estimation, where the number of objects inside a subregion is the integral of the density map over that subregion, is especially promising because it preserves spatial information, which makes it useful for both counting and localization (detection and tracking). With the power of deep convolutional neural networks (CNNs) the count- ing performance has improved steadily. The goal of this paper is to evaluate density maps generated by density estimation methods on a variety of crowd analysis tasks, including counting, detec- tion, and tracking. Most existing CNN methods produce density maps with resolution that is smaller than the original images, due to the downsample strides in the convolution/pooling operations. To produce an original-resolution density map, we also evaluate a classical CNN that uses a sliding window regressor to predict the density for every pixel in the image. We also consider a fully convolutional (FCNN) adaptation, with skip connections from lower convolutional layers to compensate for loss in spatial information during upsampling. In our experiments, we found that the lower-resolution density maps sometimes have better counting performance. In contrast, the original-resolution density maps improved localization tasks, such as detection and tracking, compared to bilinear upsampling the lower-resolution density maps. Finally, we also propose several metrics for measuring the quality of a density map, and relate them to experiment results on counting and localization.
A Survey of Recent Advances in CNN-based Single Image Crowd Counting and Density Estimation
Vishwanath A. Sindagi, Vishal M. Patel
Abstract
Estimating count and density maps from crowd images has a wide range of applications such as video surveillance, traffic monitoring, public safety and urban planning. In addition, techniques developed for crowd counting can be applied to related tasks in other fields of study such as cell microscopy, vehicle counting and environmental survey. The task of crowd counting and density map estimation is riddled with many challenges such as occlusions, non-uniform density, intra-scene and inter-scene variations in scale and perspective. Nevertheless, over the last few years, crowd count analysis has evolved from earlier methods that are often limited to small variations in crowd density and scales to the current state-of-the-art methods that have developed the ability to perform successfully on a wide range of scenarios. The success of crowd counting methods in the recent years can be largely attributed to deep learning and publications of challenging datasets. In this paper, we provide a comprehensive survey of recent Convolutional Neural Network (CNN) based approaches that have demonstrated sig- nificant improvements over earlier methods that rely largely on hand-crafted representations. First, we briefly review the pioneering methods that use hand-crafted representations and then we delve in detail into the deep learning-based approaches and recently published datasets. Furthermore, we discuss the merits and drawbacks of existing CNN-based approaches and identify promising avenues of research in this rapidly evolving field.
WiFi tracking of pedestrian behavior
Andreea-Cristina Petre , Cristian Chilipirea, Mitra Baratchi, Ciprian Dobre, Maarten van Steen
Summary
Tracking pedestrian behavior is receiving increasingly more attention. Various techniques have been used so far, yet tracking through WiFi seems to be the most popular one. This popularity comes from the ubiquity of modern smartphones, of which it is known that most have their WiFi enabled all the time. In this chapter we concentrate exclusively on how this WiFi tracking works, and explain its potentials and pitfalls. Special attention is given to the quality of data from WiFi scanning devices, and how this data can, and should be cleaned up before attempts at extracting information from sets of detected devices. As an illustration of the power of WiFi tracking, we also briefly discuss a few recent results from gathering WiFi data from a large event that attracted over 100,00 people spread across three days.
Binary Quadratic Programing for Online Tracking of Hundreds of People in Extremely Crowded Scenes
Afshin Dehghan, Member, IEEE, and Mubarak Shah, Fellow, IEEE
Abstract
Multi-object tracking has been studied for decades. However, when it comes to tracking pedestrians in extremely crowded scenes, we are limited to only few works. This is an important problem which gives rise to several challenges. Pre-trained object detectors fail to localize targets in crowded sequences. This consequently limits the use of data-association based multi-target tracking methods which rely on the outcome of an object detector. Additionally, the small apparent target size makes it challenging to extract features to discriminate targets from their surroundings. Finally, the large number of targets greatly increases computational complexity which in turn makes it hard to extend existing multi-target tracking approaches to high-density crowd scenarios. In this paper, we propose a tracker that addresses the aforementioned problems and is capable of tracking hundreds of people efficiently. We formulate online crowd tracking as Binary Quadratic Programing. Our formulation employs target’s individual information in the form of appearance and motion as well as contextual cues in the form of neighborhood motion, spatial proximity and grouping constraints, and solves detection and data association simultaneously. In order to solve the proposed quadratic optimization efficiently, where state-of art commercial quadratic programing solvers fail to find the answer in a reasonable amount of time, we propose to use the most recent version of the Modified Frank Wolfe algorithm, which takes advantage of SWAP-steps to speed up the optimization. We show that the proposed formulation can track hundreds of targets efficiently and improves state-of-art results by significant margins on eleven challenging high density crowd sequences.
Crowd Abnormal Behavior Detection Based on Machine Learning
Dongping Zhang, Huailiang Peng, Yu Haibin and Yafei Lu
Abstract
The detection of abnormal behavior is an important area of research in computer vision and is also driven by a wide of application domains, such as intelligent video surveillance. However, there are few detection algorithms to recognize abnormal behavior in crowds. This study proposed a novel method which can detect whether the crowd is abnormal or not in particular scene, such as stampede, fight and panic. For this purpose, a kind of feature extraction and description scheme has been put forward for particle flow information about crowd motion applying to space-time features cubes. The detection algorithm combined with space-time feature cubes and competitive neural network model is proposed to detect abnormal events in global region. The experimental results show that our approach achieves superior performance to abnormal behavior detection in crowds.
A Novel Wireless Sensor Network Architecture for Crowd Disaster Mitigation
Maneesha V. Ramesh, Anjitha S. and Rekha P.
Abstract
Disasters aroused due to dynamic movement of large, uncontrollable crowds are ever increasing. The inherent real-time dynamics of crowd need to be tightly monitored and alerted to avoid such disasters. Most of the existing crowd monitoring systems is difficult to deploy, maintain, and dependent on single component failure. This research work proposes novel network architecture based on the key technologies of wireless sensor network and mobile computing for the effective prediction of causes of crowd disaster particularly stampedes in the crowd and thereby alerting the crowd controlling station to take appropriate actions in time. In the current implemented version of the proposed architecture, the smart phones act as wireless sensor nodes to estimate the probability of occurrence of stampede using data fusion and analysis of embedded sensors such as tri-axial accelerometers, gyroscopes, GPS, light sensors etc. The implementation of the proposed architecture in smart phones provides light weight, easy to deploy, context aware wireless services for effective crowd disaster mitigation.
Crowd Management with RFID & Wireless Technologies
Mohammad Yamin, Yasser Ades
Abstract
The recent spread of communicable diseases like swine flu, disasters like stampedes and ongoing security issues have made management of large crowded events more critical than ever before. Managing large crowds is a very complex, challenging and costly exercise. Many of the problems encountered in crowd management can be minimized by the use of RFID and other wireless technologies. These technologies are already being used in managing and administering many activities of daily life. However, the effectiveness of these technologies is yet to be tested for managing dense crowds and poses a challenge to the industry. The aim of this paper is to provide a management framework for large & dense crowds. The analysis of the technological framework is done with help of Hajj & Kumbh case studies. The research is industrial in nature and we hope that it would help the organizers of crowded events and law and order enforcement agencies.
CROWD CONTROL SYSTEM USING IR TRANSMITTER AND RECEIVER
Yashashree Shelke, Vrushali Patil, Sayali Desale, R.S. Jagale
Abstract
An efficient crowd control system is needed for safety of lives, property, time and economy. Crowd control system presents a design and implementation of low cost, low power consummated and more reliable and an infrared based intelligent crowd control system. The system contains Infrared transmitters and receiver. The basic concept of IR (Infrared) obstacle detection is to transmit the IR signal (radiation) in a direction and signal is received at the IR receiver when the IR radiation bounces back from a surface of the object. The system can response rapidly with violation of crowd limit. System describes highly accurate crowd control system using infrared communication. Proposed system achieves high accuracy and more efficiency at four way terminals. In every direction the road will consist of an IR transmitter-receiver pair at a certain distance. When crowd will be heavy in one particular direction during emergency situation it will indicate the administrator by sending message. So the heavy crowd can be routed to other route by preventing the stampede.
CROWD VOLUME ESTIMATION USING PHOTOGRAMMETRIC TECHNIQUES
H. Attya, A. Habib, I. Detchev, A. Rawabdeh
Abstract
Incidents caused by human crowds could occur in various venues and under different circumstances, the most common being sport events, festivals, and religious events. Starting as early as the nineteenth century, research efforts have been geared towards crowd behaviour monitoring strategies especially in the fields of emergency and safety management. Attempts have been made also as recent as the mid and late nineties of the last century to use computer graphics in crowd modeling and simulation. Although crowd simulation is widely applied in several fields, research related to the derivation of quantitative crowd information is quite limited and is mainly focused on crowd volume using image processing and computer vision techniques. There were some attempts in the last two decades of the twentieth century to employ real time close-range photogrammetry in pedestrian detection and counting. However, these methods are hardly being used in high-density crowd monitoring because of the extreme difficulty in individual detection and tracking under these conditions. This paper provides a conceptual framework for the utilization of close-range photogrammetry to estimate crowd volume using a low-cost digital camera. The framework starts by developing a three dimensional model of the site in question prior to its observation in the presence of a crowd. This model will be used later to geo-reference the collected images from a dynamic camera system. The 3D model together with the geo-referencing parameters of the collected imagery will be finally used to derive crowd volume parameters. Preliminary results of the developed system will be illustrated together with the plans for the implementation of the proposed framework.
Crowd Density Estimation based on Improved Harris & OPTICS Algorithm
Cheng Xu , Hong Bao , Lulu Zhang , Ning He
Abstract
In this paper, we propose a method to estimate crowd density using improved Harris and Optics Algorithms. We pre-processed the raw images at first and the corner features of the crowd were detected by the improved Harris algorithm, then the formed density point data were used to analyze the corner characters of crowd density by the optics density clustering theory. This theory is related to the distribution of the feature points where the crowd density is estimated by the machine learning algorithm.We used a standard database PETS2009 to do the experiments in this paper and the self-shooting datasets to illustrate the effectiveness of our method. The proposed approach has been tested on a number of image sequences. The results show that our approach is superior to other methods including the original Harris algorithm. Our method improves the efficiency of estimation and has a significant impact on preventing the accidents on crowd area with high density.
Detection and Simulation of Dangerous Human Crowd Behavior
Barbara Krausz
Abstract
Tragically, gatherings of large human crowds quite often end in crowd disasters such as the recent catastrophe at the Loveparade 2010. In the past, research on pedestrian and crowd dynamics focused on simulation of pedestrian motion. As of yet, however, there does not exist any automatic system which can detect hazardous situations in crowds, thus helping to prevent these tragic incidents.
In the thesis at hand, we analyze pedestrian behavior in large crowds and observe char- acteristic motion patterns. Based on our findings, we present a computer vision system that detects unusual events and critical situations from video streams and thus alarms security personnel in order to take necessary actions. We evaluate the system’s perfor- mance on synthetic, experimental as well as on real-world data. In particular, we show its effectiveness on the surveillance videos recorded at the Loveparade crowd stampede. Since our method is based on optical flow computations, it meets two crucial prerequi- sites in video surveillance: Firstly, it works in real-time and, secondly, the privacy of the people being monitored is preserved.
In addition to that, we integrate the observed motion patterns into models for simulat- ing pedestrian motion and show that the proposed simulation model produces realistic trajectories. We employ this model to simulate large human crowds and use techniques from computer graphics to render synthetic videos for further evaluation of our auto- matic video surveillance system.
Crowd Density Estimation Based on Optical Flow and Hierarchical Clustering
Aravinda S. Rao, Jayavardhana Gubbi, Slaven Marusic, Paul Stanley and Marimuthu Palaniswami
Abstract
Crowd density estimation has gained much atten- tion from researchers recently due to availability of low cost cameras and communication bandwidth. In video surveillance applications, counting people and creating a temporal profile is of high interest. Surveillance systems face difficulties in detecting motion from the scene due to varying environmental conditions and occlusion. Instead of detecting and tracking individual person, density estimation is an approximate method to count people. The approximation is often more accurate than individual tracking in occluded scenarios. In this work, a new technique to estimate crowd density is proposed. A block-based dense optical flow with spatial and temporal filtering is used to obtain velocities in order to infer the locations of objects in crowded scenarios. Furthermore, a hierarchical clustering is employed to cluster the objects based on Euclidean distance metric. The Cophenetic correlation coefficient for the clusters highlighted the fact that our preprocessing and localizing of object movements form hierarchical clusters that are structured well with reasonable accuracy without temporal post-processing.
Automatically Detecting the Small Group Structure of a Crowd
Weina Ge, Robert T. Collins, Barry Ruback
Abstract
Recent work on computer vision analysis of crowds tends to focus on robustly tracking individuals through the crowd or on analyzing the overall pattern of flow. Our work seeks a deeper analysis of social behavior by identifying the small group structure of crowds, forming the basis for mid-level activity analysis at the granularity of human social groups. Building upon state-of-the-art algorithms for pedestrian de- tection and multi-object tracking, and inspired by social science models of human collective behavior, we automat- ically detect small groups of individuals who are traveling together. These groups are discovered using a bottom-up hierarchical clustering approach that compares sets of in- dividuals based on a generalized, symmetric Hausdorff dis- tance defined with respect to pairwise proximity and veloc- ity. We validate our results quantitatively and qualitatively on videos of real-world pedestrian scenes. Where human- coded ground truth is available, we find substantial statisti- cal agreement between our results and the human-perceived small group structure of the crowd.
Low Level Crowd Analysis Using Frame-Wise Normalized Feature For People Counting
Hajer Fradi, Jean-Luc Dugelay
Abstract
People counting is a crucial component in visual surveillance mainly for crowd monitoring and management. Recently, significant progress has been made in this field by using features regression. In this context, perspective distortions have been frequently studied, however, crowded scenes remain particularly challenging and could deeply affect the count be- cause of the partial occlusions that occur between individuals. To address these challenges, we propose a people counting approach that harness the advantage of incorporating an uniform motion model into Gaussian Mixture Model (GMM) background subtraction to obtain high accurate foreground segmentation. The counting is based on foreground measurements, where a perspective normalization and a crowd measure-informed corner density are introduced with foreground pixel counts into a single feature. Afterwards, the correspondence between this frame- wise feature and the number of persons is learned by Gaussian Process regression. Experimental results demonstrate the benefits of integrating GMM with motion cue, and normalizing the proposed feature as well. Also, by means of comparisons to other feature-based methods, our approach has been experimentally validated showing more accurate results.
Crowd Density Estimation Based on Texture Feature Extraction
Bobo Wang, Hong Bao, Shan Yang, and Haitao Lou
Abstract
As we know, feature extraction has an important role in crowd density estimation. In our paper, we introduce a new texture feature called Tamura, which is usually used in image retrieval algorithms. On the other hand, the time consuming is another issue that must be considered, especially for the real-time application of the crowd density estimation. In most methods, multiple features with high dimension such as the gray level co-occurrence matrix (GLCM) are used to construct the input feature vector, which will decrease the performance of the whole method. In order to solve the problem, we use Principal Component Analysis (PCA) method, which can obtain the mainly information of the feature using less dimension features. In the end, we use the Support Vector Machine (SVM) for estimating the crowd density. Experiments demonstrate that our method can generate high accuracy at low computational cost compared with other existing methods.
Single-Image Crowd Counting via Multi-Column Convolutional Neural Network
Yingying Zhang Desen Zhou Siqin Chen Shenghua Gao Yi Ma
Abstract
This paper aims to develop a method than can accurately estimate the crowd count from an individual image with ar- bitrary crowd density and arbitrary perspective. To this end, we have proposed a simple but effective Multi-column Con- volutional Neural Network (MCNN) architecture to map the image to its crowd density map. The proposed MCNN al- lows the input image to be of arbitrary size or resolution. By utilizing filters with receptive fields of different sizes, the features learned by each column CNN are adaptive to varia- tions in people/head size due to perspective effect or image resolution. Furthermore, the true density map is comput- ed accurately based on geometry-adaptive kernels which do not need knowing the perspective map of the input image. S- ince exiting crowd counting datasets do not adequately cov- er all the challenging situations considered in our work, we have collected and labelled a large new dataset that includes 1198 images with about 330,000 heads annotat- ed. On this challenging new dataset, as well as all existing datasets, we conduct extensive experiments to verify the ef- fectiveness of the proposed model and method. In partic- ular, with the proposed simple MCNN model, our method outperforms all existing methods. In addition, experiments show that our model, once trained on one dataset, can be readily transferred to a new dataset.
Design and Analysis of Crowd Sized Estimation Techniques
Jugal Kishor Gupta, S K Gupta
Abstract
This paper considers the different technique of estimation of crowd densities, an important part of the problem of automatic crowd monitoring and control. A new technique based on texture description of the images of the area under surveillance is proposed.
An Approach to Detect Crowd Panic Behavior using Flowbased Feature
Hao, Yu, Xu, Zhijie, Wang, Jing, Liu, Ying and Fan, Jiulun
Abstract
With the purpose of achieving automated detection of crowd abnormal behavior in public, this paper discusses the category of typical crowd and individual behaviors and their patterns. Popular image features for abnormal behavior detection are also introduced, including global flow based features such as optical flow, and local spatio-temporal based features such as Spatio-temporal Volume (STV). After reviewing some relative abnormal behavior detection algorithms, a brand- new approach to detect crowd panic behavior has been proposed based on optical flow features in this paper. During the experiments, all panic behaviors are successfully detected. In the end, the future work to improve current approach has been discussed.
Predicting Social Density In Mass Events To Prevent Crowd Disasters
Bernhard Anzengruber, Danilo Pianini, Jussi Nieminen and Alois Ferscha
Abstract
Human mobility behavior emerging in social events involving huge masses of individuals bears potential hazards for irrational social densities. We study the emergence of such phenomena in the context of very large public sports events, analyzing how individual mobility decision making induces undesirable mass effects. A time series based approach is followed to predict mobility patterns in crowds of spectators, and related to the event agenda over the time it evolves. Evidence is collected from an experiment conducted in one of the biggest international sports events (the Vienna city marathon with 40.000 actives and around 300.000 spectators). A smartphone app has been developed to voluntarily engage people to provide mobility data (1503 high-quality GPS traces and 1092694 Bluetooth relations have been collected), based on which prediction analysis has been performed. Using this data as training set, we compare density estimation approaches and evaluate them based on their forecasting precision. The most promising approach using Support Vector Regression (SMOreg) achieved prediction accuracies below 2 (root-mean-squared deviation) when compared to actual evidenced density distributions for a 12 minute forecasting interval.
Real-time Monitoring for Crowd Counting using Video Surveillance and GIS
Hongquan Song, Xuejun Liu, Xingguo Zhang, Jiapei Hu
Abstract
In public venues, crowd size is a key indicator of crowd safety and stability. Monitor the people number and crowd density levels are important scientific research topics. In this paper, we present a framework that will enable real-time crowd counting and spatial-temporal analysis for the crowd of the monitoring region. Firstly, we obtain crowd counting models for each camera by statistics regression methods using sample data. Secondly, we integrate video surveillance system and geographic information system (GIS) for capturing, managing, analyzing and displaying all forms of geographically referenced camera information, such as location, monitor area, and real-time crowd counting data, etc. And then, we combine image processing with crowd counting models to estimate people number and crowd density of monitoring areas. Finally, we implement a system for real-time crowd counting based on video surveillance system and GIS. We can acquire real-time data of people number and crowd density levels for each camera, and display them by the way of map and curves. Also, we can retrieve history data and analyze them by spatial analysis tools. The experiment shows that this system can provide early warning information and scientific basis for safety and security decision making.
Crowd Counting Mapping to Make a Decision
Enas Faisal, Azzam Sleit, Rizik Alsayyed
Abstract
Congestion typically occurs when the number of crowds exceeds the capacity of facilities. In some cases, when buildings have to be evacuated, people might be trapped in congestion and cannot escape from the building early enough which might even lead to stampedes. Crowd Congestion Mapping (CCM) is a system that enables organizations to find information about the crowd congestion in target places. This project provides the ability to make the right decision to determine the reasons that led to that and to do the appropriate procedures to avoid this from happening again by optimizing locations and dimensions of the emergency exits less congested path on the target places. The system collects crowd congestion data from the locations and makes it available to corporations via target map. The congestion is plotted on target place map, for example, the red line for highly congested location, the pink line for mildly congested location and green line for free flow of humans in the location.
Counting People in a Crowd Using Viola-Jones Algorithm
A. Alharbi, A. Aloufi, E. Hamawi, F. Alqazlan, S. Babaeer and F. Haron
Abstract
Counting the number of people in crowded areas such as the Masa’a of Al-Masjid Al-Haram has become a necessity. This is due to the increasing number of people performing Hajj or Umrah. In this paper, we propose a crowd counting system for the Masa’a using image processing techniques. The system detects the number of faces using the Viola-Jones detector then count the number of detected objects. The results show that the accuracy of the algorithm depends on the crowd density level, which are 77%, 82% and 79%, for low, medium and high density, respectively. The relatively low accuracy was due to the women wearing niqab, the face cover (usually black) since it is difficult to distinguish between a covered face from the back of the head. The system keeps the count results (i.e. total people heading to Safa or Marwah) in a database and presents the stored information in a histogram so that the authority is able to study the pattern of crowd flow and in case of overflow the system shows an alert window to warn the authorities of this dangerous situation in order to effectively manage the crowd at the Masa’a.
Crowd Escape Behavior Detection and Localization
Based on Divergent CentersChun-Yu Chen and Yu Shao
Abstract
In this paper, we propose a novel framework for anomalous crowd behavior detection and localization by introducing divergent centers in intelligent video surveillance systems. In this paper, the scheme proposed can deal with this problem by modeling the crowd motion obtained from the optical flow. The obtained magnitude, position and direction are used to construct the motion model. The method of the weighted velocity is applied to calculate the motion velocity. People usually instinctively escape from a place where abnormal or dangerous events occur. Based on this inference, a novel algorithm of detecting divergent centers is proposed: divergent centers indicate possible places where abnormal events occur. The proposed algorithm of detect divergent centers can identify more than one divergent center by analyzing the intersections of vectors, and this algorithm consist of the distance segmentation method and the nearest neighbor search. The performance of our method is validated in a number of experiments on public data sets.
Single-Image Crowd Counting via Multi-Column Convolutional Neural Network
Yingying Zhang Desen Zhou Siqin Chen Shenghua Gao Yi Ma
Abstract
This paper aims to develop a method than can accurately estimate the crowd count from an individual image with ar- bitrary crowd density and arbitrary perspective. To this end, we have proposed a simple but effective Multi-column Con- volutional Neural Network (MCNN) architecture to map the image to its crowd density map. The proposed MCNN al- lows the input image to be of arbitrary size or resolution. By utilizing filters with receptive fields of different sizes, the features learned by each column CNN are adaptive to varia- tions in people/head size due to perspective effect or image resolution. Furthermore, the true density map is comput- ed accurately based on geometry-adaptive kernels which do not need knowing the perspective map of the input image. S- ince exiting crowd counting datasets do not adequately cov- er all the challenging situations considered in our work, we have collected and labelled a large new dataset that includes 1198 images with about 330,000 heads annotat- ed. On this challenging new dataset, as well as all existing datasets, we conduct extensive experiments to verify the ef- fectiveness of the proposed model and method. In partic- ular, with the proposed simple MCNN model, our method outperforms all existing methods. In addition, experiments show that our model, once trained on one dataset, can be readily transferred to a new dataset.
Spatio-temporal Texture Modelling for Real-time Crowd Anomaly Detection
Jing Wang and Zhijie Xu, Visualisation, Interaction and Vision Research Group, University of Huddersfield
Abstract
With the rapidly increasing demands from surveillance and security industries, crowd behaviour analysis has become one of the hotly pursued video event detection frontiers within the computer vision arena in recent years. This research has investigated innovative crowd behaviour detection approaches based on statistical crowd features extracted from video footages. In this paper, a new crowd video anomaly detection algorithm has been developed based on analysing the extracted spatio-temporal textures. The algorithm has been designed for real-time applications by deploying low-level statistical features and avoiding complicated machine learning and recognition processes. In the experiments, the system has been proved as a valid solution for detecting anomaly behaviours without strong assumptions on the nature of crowds, for example, subjects and density. The developed prototype shows improved adaptability and efficiency against chosen benchmark systems.